Steep 5.6.0
The new version of my scientific workflow management system Steep has just been released. New features include automatic retrying, the possibility to deploy multiple agents, as well as an optimised scheduling algorithm.
Steep 5.6.0 also comes with a few other improvements and fixes (see complete list below). The version has been thoroughly tested in practise over the last couple of months.
Steep is a scientific workflow management system that can execute data-driven workflows in the Cloud. It is very well suited to harness the possibilities of distributed computing in order to parallelise work and to speed up your data processing workflows, no matter how complex they are and regardless of how much data you need to process. Steep is an open-source software developed at Fraunhofer IGD. You can download the binaries and the source code of Steep from its GitHub repository.
Automatic retrying
It is now possible to specify retry policies to define how often a certain service or workflow action should be re-executed in case of an error. The feature is best explained with an example:
- type: execute
service: cp
inputs:
- id: input_file
var: input_file
outputs:
- id: output_file
var: output_file
retries:
maxAttempts: 5
delay: 1s
exponentialBackoff: 2
maxDelay: 10s
The example shows an execute action
that copies an input_file
to an output_file
. The retry policy
(attribute retries
) specifies that the action should be re-executed if the
copy process fails. The maxAttempts
attribute defines the maximum number of
executions including the initial attempt. In this example, Steep will run the
action one time and then retry it up to four times (1 + 4 = 5). Between each
attempt, Steep will wait at least 1 second, which is specified by the delay
parameter. Since the exponentialBackoff
factor is set to 2, this delay will
double on each attempt. The maximum delay between two attempts is 10 seconds
in this example.
You can specify retry policies in the service metadata or in an execute action. Read more about this feature in Steep’s documentation.
Deploy multiple agents
In previous versions, each Steep instance contained exactly one agent. This meant that if you wanted to run multiple process chains in parallel on the same machine, you had to start Steep more than once.
The new version 5.6.0 allows you to specify how many agents should be deployed
per Steep instance through the new steep.agent.instances
configuration item. This
can help you make use of full parallelism without wasting resources.
Optimised scheduling algorithm
I’ve invested a lot of work into optimising Steep’s scheduling algorithm to make it much more scalable. The new version caches responses from remote agents in order to avoid having to send too many messages over the event bus. Agents that are known to be busy as well as those that definitely do not support a given required capabilities set will be skipped during scheduling.
In addition, the scheduling algorithm now does not stop anymore if it cannot assign a process chain to an agent. Instead, it continues with the other process chains and agents. This improves the scheduling throughput (i.e. it increases the number of process chains assigned to an agent in one scheduling step).
Steep now also does not unnecessarily run multiple scheduling lookups in parallel. This saves resources and further improves scalability. I’ve also added a MongoDB compound index to speed up fetching process chains.
Finally, Steep now selects the best required capabilities by the total count of remaining process chains. This makes sure process chains are distributed more evenly to agents supporting similar capabilities, which can reduce the overall runtime of workflows.
The following figures taken from my upcoming paper on the improved scheduling algorithm show the improvements.
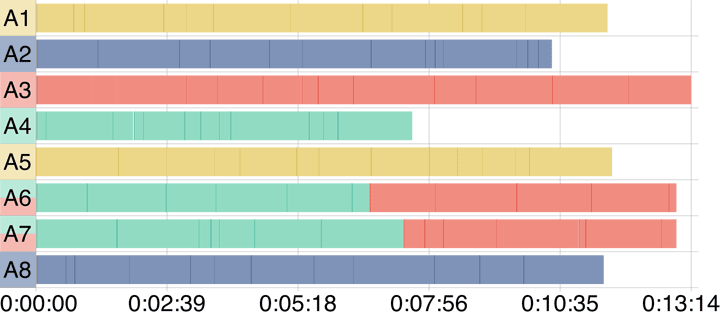
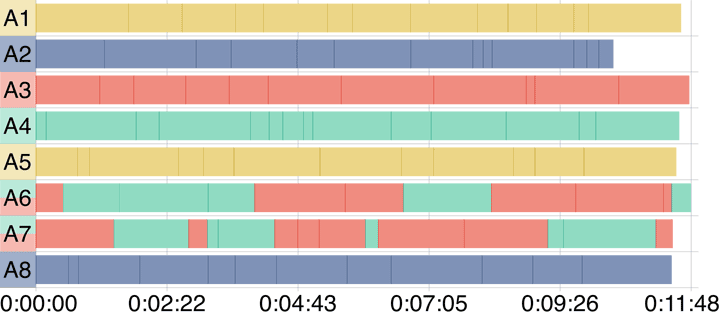
The new algorithm reduces the total runtime of this example workflow by about one and a half minutes.
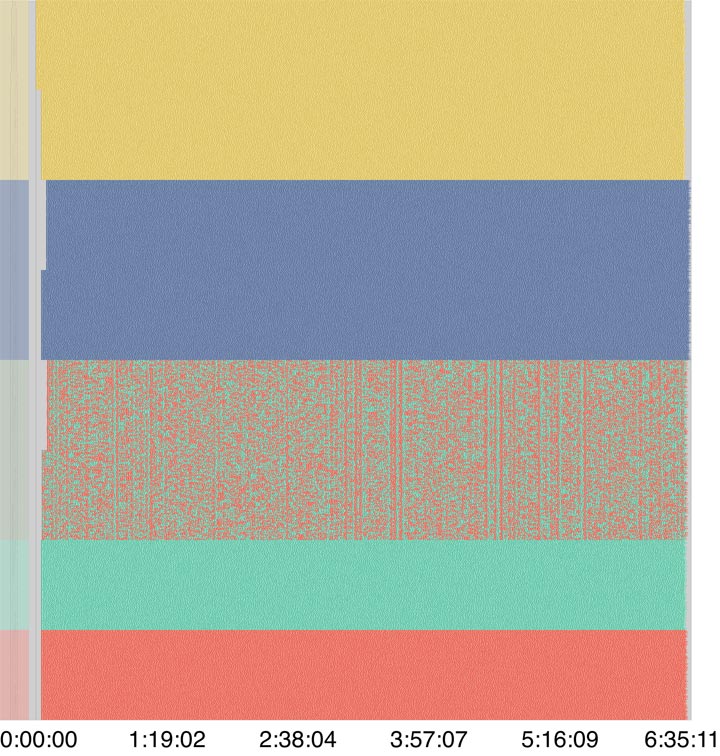
The improved scheduler is very scalable. It is able to successfully execute 300,000 process chains on 1,000 agents.
Image source: Krämer, M. (2020). Efficient scheduling of scientific workflow actions in the Cloud based on required capabilities. (Submitted to Data Management Technologies and Applications)
Other new features
Besides the features mentioned above, the new version contains the following improvements:
- The time each scheduling step took will now be logged
- Multiple orphaned VMs will now be deleted in parallel
- The default value of the store flag will not be included in serialized workflows anymore
- Additional Prometheus metrics are now exposed through the HTTP interface:
- Number of process chains executed by the scheduler
- Number of retries performed by the local agent (per service ID)
Maintenance
- Update UI dependencies
- Remove unnecessary log messages
Bug fixes
- Pull alpine docker image before running unit tests
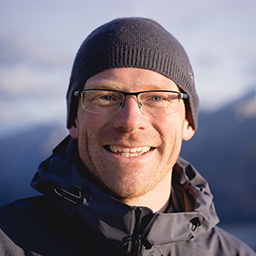
Posted by Michel Krämer
on 14 November 2020
Next post
Steep 5.7.0
I’ve just released a new version of my scientific workflow management system Steep. It introduces live process chain logs, improved VM management, and many other new features. This post summarises all changes.
Previous post
Fast and scalable point cloud indexing
In our paper, we introduce a system for fast, scalable indexing of arbitrarily sized point clouds based on a task-parallel computation model. We evaluate our system in two experiments processing datasets with several billion points.
Related posts
Steep - Run Scientific Workflows in the Cloud
I’m thrilled to announce that the workflow management system I’ve been working on for the last couple of years is now open-source! Read more about Steep and its features in this blog post.
Steep 5.8.0
I’m thrilled to announce the new version of the scientific workflow management system Steep. This release contains many features including the possibility to resume process chains after a scheduler instance has crashed.
Steep 6.0.0
The new version of the scientific workflow management system contains many new features including an improved workflow syntax, better parallelization, workflow priorities, and full-text search. It also fixes a few bugs.